One of the most influential economics of innovation papers from the last decade is “Are Ideas Getting Harder to Find” by Bloom, Jones, Van Reenen, and Webb, ultimately published in 2020 but in earlier draft circulation for years. While the paper is ostensibly concerned with testing a prediction of some economic growth models, it’s broader fame is attributable to it’s documentation of a striking fact: across varied domains, the R&D efforts necessary to eke out technological improvement keep getting higher. Let’s take a look at their evidence, as well as some complementary evidence from other papers.
Moore’s Law
Bloom and coauthors start with Moore’s law, the observation that for the last half century, the number of transistors that can fit on an integrated circuit doubles every two years.
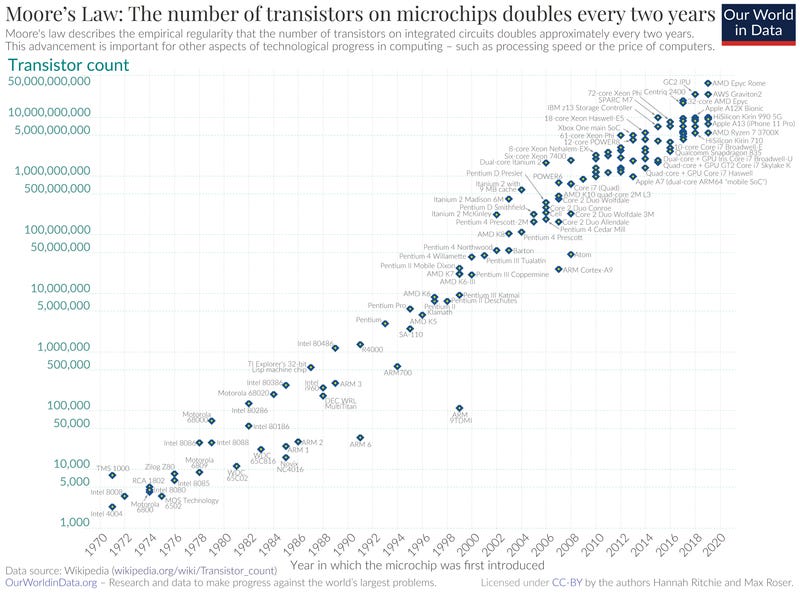
Each doubling of circuits is the fruit of human ingenuity. What Bloom and coauthors show is that the amount of human minds we have to throw at this problem to keep up this pace of doubling keeps rising:
In the figure above, the green line with the axis on the right is annual spending on semiconductor R&D divided by the wages of researchers; effectively, it’s the number of brains you can buy to throw at this problem if you expend your entire R&D budget on hiring (hence, “effective” number of researchers). While the rate of progress has been steady at 35% per year, the effective number of researchers has grown nearly 20-fold since 1971. It gets harder and harder to achieve a doubling of chips per integrated circuit on this schedule.
Crop Yields
Next, Bloom and coauthors turn to agriculture. Agriculture is a nice setting for studying innovation because agricultural products have been mostly unchanged for decades - maybe even centuries. While a phone today is not the same thing as a phone from fifty years ago, an ear of corn today is more or less the same as an ear of corn from fifty years ago. So it’s relatively easy to measure long-run technological change in agriculture. Like Moore’s law, the increase in annual yields across major crops has been remarkably consistent for half a century, on the whole (it bounces around more than semiconductors because of the weather).
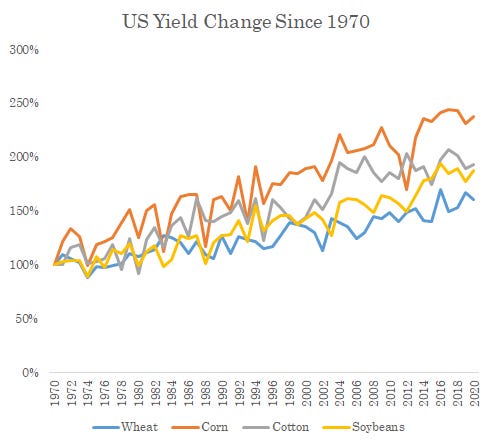
Yet once again, those gains are costing us more and more. In the figure below we have the annual growth rate of yields of different crops, in blue, and again estimates of the number of effective researchers working on the problem of increasing yields in green. The top green line is a “narrow” measure of yield growth research, focusing on the growth of R&D effort devoted to breeding or engineering better crops. The lower green line is a “broad” measure of yield growth research, adding in research on things like chemical pesticides and fertilizers.
For corn and soybeans, the pattern is just like with Moore’s law. No matter how you slice it, the scale of R&D resources devoted to improving yields has increased 6-fold (if considered broadly) or more than 20-fold (if considered narrowly), with no concomitant increase in yield growth. However, for cotton and wheat, there are long periods where R&D resources remained roughly constant, and yet were able to maintain constant yield growth. We’ll see elsewhere that this does happen some of the time.
Before we move on, I can’t help pointing out a weird parallel between agricultural yield growth and Moore’s law. Just as Moore’s law is about packing transistors more densely onto circuit boards, the growth in agricultural yields is mostly about packing plants more densely onto farmland. At least, this is the case for corn (it may well be for other crops as well, but I haven’t seen data). The figure below plots changes in corn bushels per plant (in blue), plants per acre (in orange), and bushels per acre (in grey) in the state of Iowa (where I live). While a corn plant in 1963 yielded basically the same number of bushels as a plant in 2020, yield has more than doubled because we’re now packing more than twice as many of those plants onto each acre.
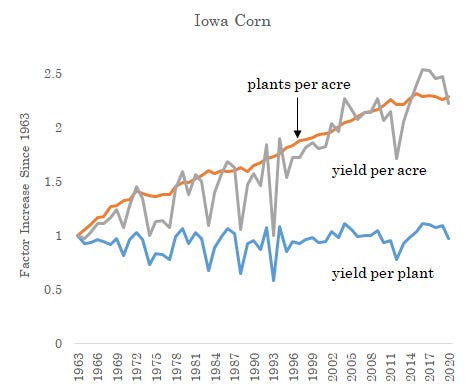
Health
Next, Bloom and coauthors look at health outcomes. In this case, rather than trying to count how many researchers or research dollars are being spent on any given disease (particularly challenging in the presence of spillovers), they measure the amount of research devoted to each disease by counting the number of publications or clinical trials related to a given disease. To measure progress against each disease, they calculate life years lost due to each disease (for a population of 100,000 people) and back out life-years saved. Below, they calculate the number of years of life saved by a given measure of R&D effort (clinical trials or journal publications).
Two observations. There are periods for which a constant supply of R&D effort does generate constant improvements, or even increasing improvements. These occur mostly before 1990. But in the long run, this seems to be another area where it takes more and more R&D effort - here measured in journal publications or clinical trials - to eke out constant improvements.
Machine Learning
In a domain not covered by Bloom and coauthors, Besiroglu (2020) is an interesting master’s thesis that compares progress in machine-learning to research effort. Besiroglu computes research effort in different machine learning domains with the number of unique authors publishing papers on these topics (in Web of Science and arXiv). Progress in machine learning can be measured on a wide variety of widely accepted benchmarks, such as accuracy classifying images in a standard dataset. Broadly speaking, increased research efforts have not yielded any visible increase in the growth rate of progress.
In the figure above, we have Besiroglu’s estimate of research effort related to computer vision at left, one example of a measure of progress on computer vision (there are actually 56 different measures for computer vision) in the middle, and a statistical model-based estimate of research productivity at right. The main take-away is clear: even though R&D resources have increased by an order of magnitude (note the figure at left has a log scale), the rate of progress has not sped up, because improvement per researcher has fallen. These results also hold for natural language processing and machine learning on graphs.
Firms At Large
This is pretty suggestive, but at the end of the day it’s quantitative case studies, and with case studies we might always be a bit worried that the cases selected are unusual. So Bloom and coauthors also extend their analysis to the much larger set of all US publicly traded firms.
It’s not that hard to compute R&D effort for all these firms - divide their R&D spending by the typical wage of scientist. The trouble here is coming up with a measure of “innovation” that is consistent across different kinds of companies doing different kinds of things. Bloom and coauthors resort to some crude measures that are plausibly linked to innovation: growth in sales, market capitalization, employment, and revenue per worker. The idea here is that a more innovative firm might create better products and services or find cost efficiencies that lead to growth along all these dimensions. These measures are crude though because many things besides innovation can affect them - a non-innovating firm that enters a new market, for example, could see growth in most of these metrics. But with thousands of observations, hopefully these omitted factors are randomly distributed among innovating and non-innovating firms, over time, so that if we just look at how our measures of “innovation” per R&D worker change over time, they won’t be misleading. If this was the only data Bloom and coauthors presented it wouldn’t be very convincing, but in concert with the other data we’ve seen, it’s more compelling I think.
Comparing growth in sales per R&D worker across two consecutive decades, they compute the change in “research productivity” for all these firms. The distribution of results is below (in blue):
The main message you should take from this is that most of the blue bars lie to the left of 1. That means growth in sales per R&D worker dropped from one decade to the next, for most firms. Note that it’s not universal though. As we saw for some crops, and for some of the time with health innovation, some firms observed an increase in research productivity over two decades.
So far all of these examples have been US-specific, so one objection might that this is actually something specific to the USA. Maybe this just reflects that fact that we’re a country that’s in decline for various reasons that are unique to us? Boeing and Hünermund (2020) replicates this part of Bloom et al. (2020) for Germany and China though and get the same flavor of results.
This data is once again computing growth in sales per effective R&D worker, for a broadly representative sample of German firms (that conduct R&D) and publicly traded Chinese firms. While the decline in research productivity is pretty similar in the USA and Germany, the decline is much higher in China.
As we’ll see, this isn’t the only evidence that innovation is getting harder in more places than just the USA.
Nations and Industries
Another crude but common measure of innovation is total factor productivity. Total factor productivity - TFP for short - is statistically estimated as the amount of quality-adjusted output that can be squeezed out of a mixed set of various inputs (e.g., capital, labor, land, energy, etc.). If you invent a new process to more efficiently make the same amount of output with less inputs, that shows up as an increase in TFP. Similarly, if you invent a new and more valuable kind of product that doesn’t take more inputs to build, that can also show up as an increase in TFP. Importantly, you can compute TFP for entire industries or economies, making it a favorite measure of innovation writ large. Note, however, that these measures can also be misleading: TFP can move for reasons unconnected to innovation. But again, in concert with the other evidence, it starts to look more compelling.
Miyagawa and Ishikawa (2019) have a working paper that uses TFP to look at how research productivity has changed over 1996-2015 for a set of Japanese manufacturing industries and for manufacturing and information services overall in Japan, France, Germany, the UK, and the US. Within Japanese industries, they find a mixed bag; some industries saw TFP growth per effective researcher rise over the period and others saw a fall. Overall, there was a decline in research productivity in Japanese industries, but not a statistically significant one.
Looking more broadly at how research productivity changed in the overall manufacturing sector of various countries, they do find declining research productivity in all five countries they study. But when they look at how research productivity in the information services sector they again find a mixed bag. In Germany, for example, TFP growth per effective R&D worker was higher in 2006-2015 than in 1996-2005.
Lastly, let’s return to Bloom and coauthors. In the USA, we can estimate total factor productivity for the entire country, as well as R&D effort, going all the way back to the 1930s. When we compare those two datasets, we see the same thing, going all the way back to the beginning. For as long as we have data, it’s taken increasing effort to sustain a constant rate of TFP growth.
So, looking at the rate of technological advance across a variety of sectors - computer chips, agricultural yields, health, and machine learning - we see a strong tendency for a constant rate of advance to be only sustainable by significantly increasing research efforts. Proxies for innovation in firms, industries, and countries find the same general tendency. The march of progress needs more and more effort to sustain it. This is not a universal rule. There are exceptions in certain fields that can sometimes go on for decades. But it does seem to be a general tendency. Innovation gets harder.
To close though, let’s consider a few potential objections to all this evidence.
Objection! Mismeasurement!
One common complaint about this exercise is that the case studies focus on the wrong things. For example, agricultural crop research is about a lot more than maximizing yields. GMO technology that makes a crop resistant to pests may not increase yield much, but it makes farming more profitable by reducing the need for some pesticides. Other agricultural crop research reduces the vulnerability of crops to extreme heat or drought; in a year without drought or heat stress, you won’t see any impact of this research. If an increasing share of research is devoted to these non-yield factors, than it might be that R&D is just as productive as ever, but we’re not measuring the correct outputs of R&D effort. You could make similar claims about the other case studies.
Totally fair. More correctly measuring the goals of research with R&D effort will probably make R&D effort look more productive. But it’s hard for me to believe the effect would be big enough to change our conclusion that innovation gets harder, as a general tendency. Looking at corn, research effort is up 6-20-fold depending on how you measure it. To have constant R&D productivity, that means the share of research that leads to better yields needs to fall by an offsetting 83-95%. It wouldn’t surprise me that the share of research devoted to yield growth has fallen over this period, but I don’t believe it’s cratered to a tiny fraction of overall effort, relative to the 1970s.
The numbers don’t look great for the other case studies either. Semiconductor research is about more than cramming transistors on circuits. But has the share of research devoted to that goal really fallen from 100% of R&D effort in 1970 to 5% in 2015? That’s the kind of change that would be needed to generate these numbers when research is actually just as productive as ever.
Objection! Growth is just linear!
Second, we might ask if it’s really appropriate to expect the rate of progress to be related to the number of scientists working on something. As a counter-example, imagine we’re talking about culinary scientists inventing new recipes. Suppose culinary scientists can come up with one new recipe per year. At one recipe per year, the growth rate of recipes is 10% per year when there are just 10 recipes, but 1% per year when there are 100. The rate of progress slows down, if the number of culinary scientists doesn’t change. Yet, in this thought experiment, we can’t really say innovation has gotten harder. It’s just that progress is constant and linear, and we’re incorrectly assuming it should be constant and exponential.
This is super reasonable. In fact it’s so reasonable that this is almost exactly what economic growth models do assume! Remember, at the beginning of this post, I said that Bloom et al. (2020) was ostensibly motivated as a test of one of the predictions of some economic growth models? This is closely related to the prediction the paper is trying to test.
The main difference between our thought experiment about culinary scientists and models of economic growth, is that the models assume the absolute increase in innovations (e.g., recipes per scientist per year) is related not to the number of scientists but the level of actual real R&D resources devoted to research. Think of this as a mix of labor and capital; scientists plus lab equipment, computers, arXiv, and everything else used to support inquiry. In terms of our example, these models assume a culinary scientist plus the same set of “culinary research tools” (a test kitchen and library of cookbooks?) would generate one new recipe per year.
There is a subtle distinction between R&D resources and R&D “effort”, as I’ve called it in this post. These models assume the same level of actual R&D resources always generate the same level of innovations. But the nature of R&D resources that a given level of R&D effort uses will change over time. The resources will improve, and come to embody technological advances themselves. Intuitively, the same level of “effort” will now go further, because it will be able to draw on faster computers, access deeper knowledge, and so on.
R&D effort in this post is measured as the effective number of researchers - that is, the number of researchers you could hire if you spent all your R&D on researchers. But that’s not what people actually do. In fact, they buy a bundle of research labor and research capital. The trick is that the capital side gets cheaper or “better” as an economy grows. Under some seemingly reasonable assumptions, all these effects balance out so that the same number of researchers, supported by capital embodying steadily better knowledge, can sustain a constant rate of technological progress. That is what is predicted by these models, and that is the prediction that is apparently falsified by all this evidence.
Let me say it one more time: under some seemingly reasonable assumptions, if you write down a model of economic growth where a constant level of real R&D resources generates a constant level of innovations, that will still lead to a constant growth rate of innovation, because R&D resources improve with the overall economy. This is the prediction Bloom et al. (2020) shows does not hold.
But I think there is another, even simpler way to respond to this critique (that we should never have expected innovation to be constant and exponential with the same number of researchers, but that it should be constant and linear). The rate of progress is what most people care about because that is what we’ve become accustomed to. It’s business as usual. We expect our computers to get twice as fast every few years because that’s how it’s been in our adult lifetimes. We expect crops to yield a couple more bushels per year, because that’s how it’s been in our lifetimes. We expect healthcare to save a few more years of life, machine learning benchmarks to be notched up, and to be a few percent richer as a society, every year, because that’s what we’re accustomed to. And what this line of work shows is that sustaining that business as usual requires steadily more effort.
If you liked this, you might like these other posts:
You might also want to subscribe!
Innovation gets (mostly) harder