Like the rest of New Things Under the Sun, this article will be updated as the state of the academic literature evolves; you can read the latest version here.
How do scientists and inventors decide what to work on? Part of it comes down to what they find personally meaningful. And that, in turn, can be informed by your specific life experiences.
We can actually see this in data, if we look in the right places. Every human life is unique, but in this article we’re going to collapse diverse life experiences down into something quite crude: binary gender. This can be a useful exercise because, as we’ll see in a minute, there are a variety of non-controversial strands of evidence that women are slightly more likely to work on some things than men, and that this may well reflect different perceptions on what questions are meaningful (likely derived from lived experience). But it’s also an exercise fraught with risks, because there may well be a variety of other factors that lead men and women to work on different topics.
For example, it turns out that the share of scientists who are women differs a lot across fields. Over 1990-2011, West et al. (2013) find that 41% of published authors in sociology are women, compared to just 14% of authors in economics. Should we read this as evidence that different life experiences lead men and women to have different interests in sociology, relative to economics? I don’t think so, as there are many other possible barriers to entry in one field relative to another: discrimination or bias, culture, access to networks, and so on.
But cognizant of these risks, we can still look at the choices people make within a specific, narrow, field. That won’t completely rule out the possibility that the choices of research question is constrained by bias and discrimination (even within a specific subfield), but it’s an informative place to start.
Gender and the Direction of Technological Innovation
Koning, Samila, and Ferguson (2021) analyze a set of ~400,000 US biomedical patents from 1976-2010. For each patent, they classify the gender of the inventors based on their names (they can match 98% of inventors with 95% confidence; note they have a binary classification of gender, which they point out is a not appropriate in all cases). They next run a sample of the text of these biomedical patents through an algorithm designed to classify academic articles into different biomedical categories. This algorithm classifies about 13% of these biomedical patents as being related to “female organs, diseases, physiologic processes, genetics, etc.” and another 13% as being related to male analogues. They also cross-check these classifications with a variety of alternative metrics: are patents classified as having a female focus more likely to be based on all-female clinical trials? Are they more likely to be related to diseases that have much higher incidence in women than men?
They find that patents where the majority of the inventors are men are more likely to patent male-focused patents and patents where the majority of the inventors are women are more likely to patent female-focused patents.
Einiö, Feng, and Jaravel (2019) find something similar using a variety of other datasets.
Via Nielsen data, they have information on a sample of households that purchase a wide range of specific consumer products, as well the barcode associated with each product. They can link these barcodes back to the manufacturing firm, which they can then link to patents owned by these firms. Finally, they can use the same strategy as Koning, Samila, and Ferguson to classify the inventors on these patents as men or women. This lets them draw a connection between the gender of inventors and the gender of the people purchasing products manufactured by the companies that own these patents. They find, indeed, that products that are disproportionately purchased by female households also have a higher share of female inventors.
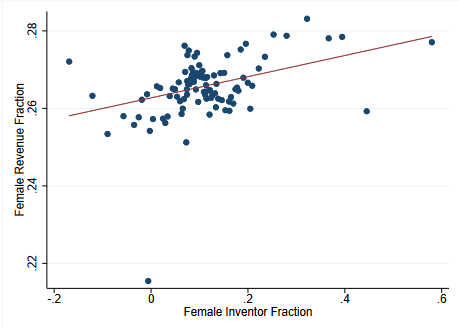
Stepping away from patents, they also use data from Crunchbase to identify startups with female founders, and use the same Nielsen data to assess the gender of purchasers of these startups’ products. They find that startups with a female founder are also more likely to sell products with a disproportionately female consumer base.
So along three different axis, we see that inventors who are women (or at least, have names traditionally given to women) are more likely to develop new products and new technologies that appeal to women, as compared to inventors who are probably men. We can see a similar effect when we look at research papers.
Gender and the Direction of Science
Koning, Samila, and Ferguson (2021) also perform a similar exercise on biomedical science. They extract data on about 2 million biomedical research articles published between 2002 and 2020 and once again classify the authors as male or female based on their names. They focus this analysis on original research published in journals whose articles tend to be highly cited by patents (because this paper’s main focus was on patents).
There’s no need to process these articles through a machine-learning algorithm to see if they focus on female or male related topics (i.e., pertain to female/male organs, diseases, physiologic processes, genetics, etc.), since the articles had already been classified into biomedical categories as part of the publication process. Again, they find articles with more women as authors are more likely to focus on female topics. The following figure is how much more likely a given article is to be female-focused, estimated with two different statistical models.
(If interested - the black estimate comes from a basic regression, controlling for journal-year and team size-year commonalities, the gray one from a model where teams are matched to all-male control teams that are observationally similar).
Nielsen et al. (2017) looks at the influence of gender on biomedical research in a different way. Rather than seeing if papers are classified as pertaining to male or female biomedical categories, Nielsen and coauthors look to see if the gender of the author affects the probability papers incorporate gender and sex analysis, defined by them as “scientific approaches that are aimed at understanding how social and behavioral differences between women, men and gender-diverse people (gender analysis) and biological differences between female and male research subjects (sex analysis) relate to health outcomes.” The GenderMed dataset identifies approximately 5,000 papers, published between 2008 and 2015, incorporating such an analysis. Over the same period, about 1.5 million papers were published on diseases for which such an analysis can be relevant, so a gender and sex analysis is a pretty rare event (3 in 1,000 papers). Nonetheless, Nielsen and coauthors find papers with more women coauthors were more likely to include such an analysis, even within a specific disease category and country.
First and Second Order Effects of Representation
These differences are never huge, but they are persistent across quite a range of evidence. It seems to me pretty likely this reflects either a relative lack of awareness or a relative lack of empathy by male scientists/inventors about the importance of these issues. That, in turn, supplies one reason why representation in science is important. If we want research to broadly reflect the priorities of society at large, and if a part of society is not represented in the research space, the above evidence suggests we’re not going to get the kind of research we want.
That’s a first order effect: if you want research related to a specific group, the chances of getting it may be higher if that group is able to participate in the research process. But there is also a second order effect. We also have some evidence that, as representation improves, everyone’s research priorities shift.
For example, let’s look again at the probability majority male inventor teams works on a female-focused biomedical patent compared to a male-focused one (left figure below). The gap between the two has been closing. Could that be because the number of female inventors has been rising (right figure below), which is increasing male awareness of female-focused research?
Nielsen et al. (2017) also look beyond the impact of female coauthors, to the broader effect of more women in a particular subfield. They find that when there are more women studying a particular disease, this also increases the probability that studies include a gender and sex analysis, independent of the composition of the team of coauthors on any individual study. In other words, a team of men is more likely to include a gender and sex analysis if they are working on a disease where more of the scientists studying the same disease are women.
That could be because as women attain positions of influence - serving as peer reviewers, grant reviewers, PhD supervisors, etc. - the rest of the field becomes more responsive to their concerns. As discussed in “Conservatism in Science” we do have evidence that scientists constrain the feasible choice of research topics for their peers through a variety of channels like this.
But it could also be that people’s awareness and empathy can change when they are around people different from themselves.
An exposure experiment?
It’s tough to experimentally vary “awareness” and “empathy” but a 2021 dissertation by Truffa and Wong look at a situation that has parallels to this. Between 1960 and 1990 76 all-male US universities went coed and began to admit women as undergraduates. Truffa and Wong look to see what happens to the research of these universities before and after they make the switch.
They identify all the academic papers (in the Microsoft Academic Graph) with authors affiliated with these universities, and then they classify papers as being related to gender if the title or abstract includes various keywords (for example “lady”, “female”, “misogyny”, “mothers”, “sexism”). Note that this approach covers all fields, not just the life sciences. A manual inspection of 100 random papers identified as being gender-related by this keyword approach finds the method works pretty well, with only about 8% of the papers identified in this way not really related to gender. They also get similarly encouraging results when they benchmark this against other techniques (for example, by training a machine-learning algorithm on gender studies papers and papers in gender-related journals; or focusing just on biological papers and comparing the results to the biomedical classification systems discussed earlier).
When these universities began to admit women, they also began to produce more research related to gender, as illustrated in the following figure1 (with the year of going coed centered at zero). The effect is small in absolute terms, but large relative to the pre-existing number of papers related to gender (just 2% in 1960 at these universities).
Why does this happen?
Well, it could have nothing to do with the changing composition of the undergraduates. Maybe these institutions had already decided to take gender more seriously, and that was reflected simultaneously in research and the choice of which students to admit. But Truffa and Wong argue the actual reason for the transition from all-male undergraduates to mixed was not so high-minded. Instead, all-male schools found they were having a harder time attracting “top boys”, who increasingly preferred to attend coed universities. So, to continue attracting “top boys” these institutions (grudgingly?) began to accept undergraduate women too. In other words, the decision to go coed does not seem to have been driven by faculty itching to take gender more seriously in their research.
So why did research begin to take gender more seriously after the schools went coed? It could be that going coed was accompanied by increased hiring of women faculty. As we’ve seen above, it shouldn’t be that surprising that institutions that hire more women will end up getting more women-centered research. Truffa and Wong do find that the share of new assistant professors who were women rose from about 13% to 17% after schools went coed. But the effect goes well beyond these new hires.
Instead, a large part of this effect seems to be driven by pre-existing faculty revising their research preferences. As the figure below shows, even incumbent male professors were more likely to do gender related research after the undergraduate body included women.
These effects were bigger at colleges that admitted more women after the change. Truffa and Wong also present some evidence about a few very concrete channels through which these changes might have occurred. For example, the number of gender-related classes increased significantly after colleges went coed, and in preparing and teaching these classes, faculty might have begun to engage more with these ideas. Second, undergraduates may sometimes participate in the research process. For example, in psychology research of this era, it was common to perform experiments with undergraduate volunteers, and a coed pool of volunteers would have made it easier to do experiments that examined gender differences. Truffa and Wong show, in psychology, the increase in gender-related papers does indeed stem from experimental papers.
The Importance of Representation
The takeaway then, is that representation matters. When a group previously excluded from research enters, it may carry with it research priorities that are better aligned with the needs of the excluded group. Fortunately, as those ideas enter the bloodstream of a research community, it looks like the priorities do get taken up more widely.
But we remain a long way from gender parity in most of research. Holman, Stuart-Fox, and Hauser (2018) examine the rate at which the gender gap in science is closing. Across 115 disciplines that publish to the PubMed or arXiv paper repositories, 87 had fewer than 45% women authors in 2016. In almost every one of these fields the share of women was increasing over time, but at the current rate in most cases it would be well over a decade for authors to come within 45%, sometimes far longer (and longer still for more senior positions). Lastly, there is no reason to believe similar dynamics don’t play out with other underrepresented groups.
Thanks for reading! If you liked this, you might also enjoy this article about some of the forces that bias science away from novelty and towards conservatism. For other related articles by me, follow the links listed at the bottom of the article’s page on New Things Under the Sun (.com). And to keep up with what’s new on the site, of course subscribe!
Technical note: There have been some controversies around this methodology (difference-in-difference with staggered treatment). In a robustness check, the paper shows they obtain basically the same results when they use a technique from Sun and Abraham (2020) designed to deal with some of these issues.
Share this post