Welcome to the final post of patent data week!
This week we’ve been working through four related posts I wrote about using patent data to measure innovation:
Tuesday: Patents (weakly) predict innovation
Wednesday: Do studies based on patents get different results?
Today’s post is meant to be the definitive one, synthesizing what we learned in the previous three days. Substack says it’s too long for email, so you might need to read this one on Substack or the website. Enjoy!
Can we learn about innovation from patent data?
This article will be updated as the state of the academic literature evolves; you can read the latest version here. You can listen to this post above, or via most podcast apps here.
The tagline for New Things Under the Sun is “What academia knows* about innovation.” But a large share of the academic literature is premised on using patents to measure innovation. And it’s not clear patents actually measure innovation. Maybe academia knows less than it thinks?
At first glance, patents seem like an ideal measure of innovation. Patents are detailed, available for a huge range of technologies, and go back in time for hundreds of years. By providing an incentive for inventors to disclose their inventions, as well as a screening system staffed by experts, they hold the promise of providing us a large scale, high quality, survey of innovation.
But patents also have some red flags too. For many years the company with the most patents per year was IBM - surely a company that innovates, but not the company most people probably think of as the most innovative company in America. Moreover, it’s not hard to find lists of important inventions that never received a patent, or patents that seem to correspond to junk.
I think the following figure1 is perhaps the best single case against using patents to measure technological progress. The figure compares the count of annual applications at the US patent office (solid line), against another common (though also flawed!) measure of technological progress, namely the ten-year average growth rate in capital utilization-adjusted total factor productivity growth (dashed line).
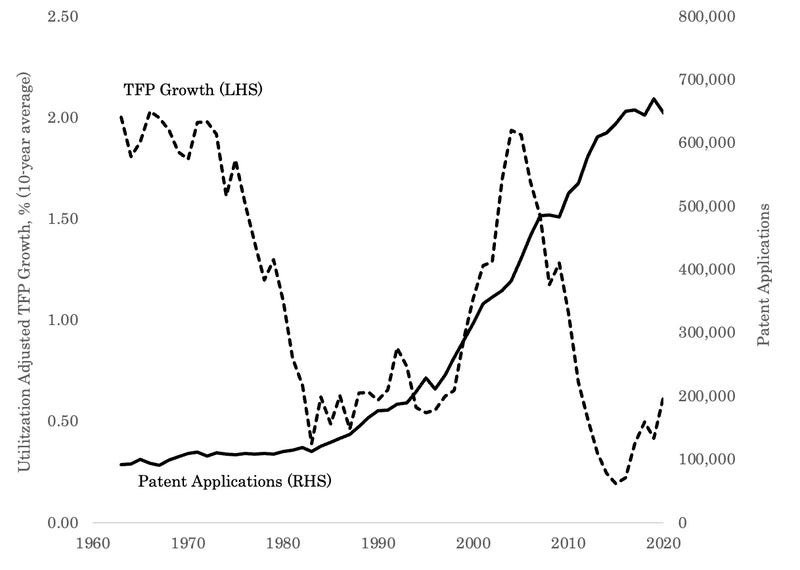
In the figure, total factor productivity growth drops sharply in the 1970s, while patent applications are unchanged. Both rise from 1980 through 2000, but then total factor productivity plunges to new lows as patent applications rise to new highs. (You obtain essentially the same pattern looking at granted patents rather than applications). Not only do the two fail to track each other, but in a raw scatterplot they are actually negatively correlated! This chart suggests patents are positively useless as a way to measure innovation. Maybe worse than useless, if they are inversely correlated with technological progress!
But I don’t think patents are useless, much less that they are worse than useless. Instead, I think patents give us a biased sample of inventive activity. Good work with patents is about understanding and adjusting for those biases. Handled well, we can learn a lot that is useful from them. That view is based on a few lines of evidence I’ve written about before.
How many inventions are patented?
Let’s start with a straightforward question: how many inventions are patented?2
Let’s start with the good news, at least for researchers who want to use patents to study innovation. Recent surveys find more than 90% of US R&D is performed by companies that have at least one patent. Patents also appear to be a pretty good measure of innovation in the pharmaceutical sector.
But other lines of evidence are less promising. In manufacturing, if we survey firms, they claim to protect about half their new products with patents and a third of their newly invented processes with patents. Another option is to use innovation prize competitions to generate lists of inventions; we can then see how many entrants or winners have associated patents. Many papers have done this, generally finding patent matches for only 10-30% of inventions (with one major exception, a study from Japan that finds nearly all prize winners have an associated patent). Another study measuring invention by the introduction of consumer products with new UPC codes (mostly in grocery and drug stores) finds about 20% of new products are introduced by firms with patents in closely related product categories. These matching approaches are probably lower bound estimates, since they miss patents that do not seem to be related to an invention, but do in fact protect them. Nonetheless, I think we can be pretty sure less than half of inventions get patented. This literature also finds a great deal of variation in the propensity to patent across different sectors.
So a minority of inventions are patented. But that’s only half the story. It tells us something about the share of inventions that are patented. But if we’re going to measure innovation with patents, we also want to know the share of patents that describe innovations. Because on that criterion, some patents are pretty questionable.
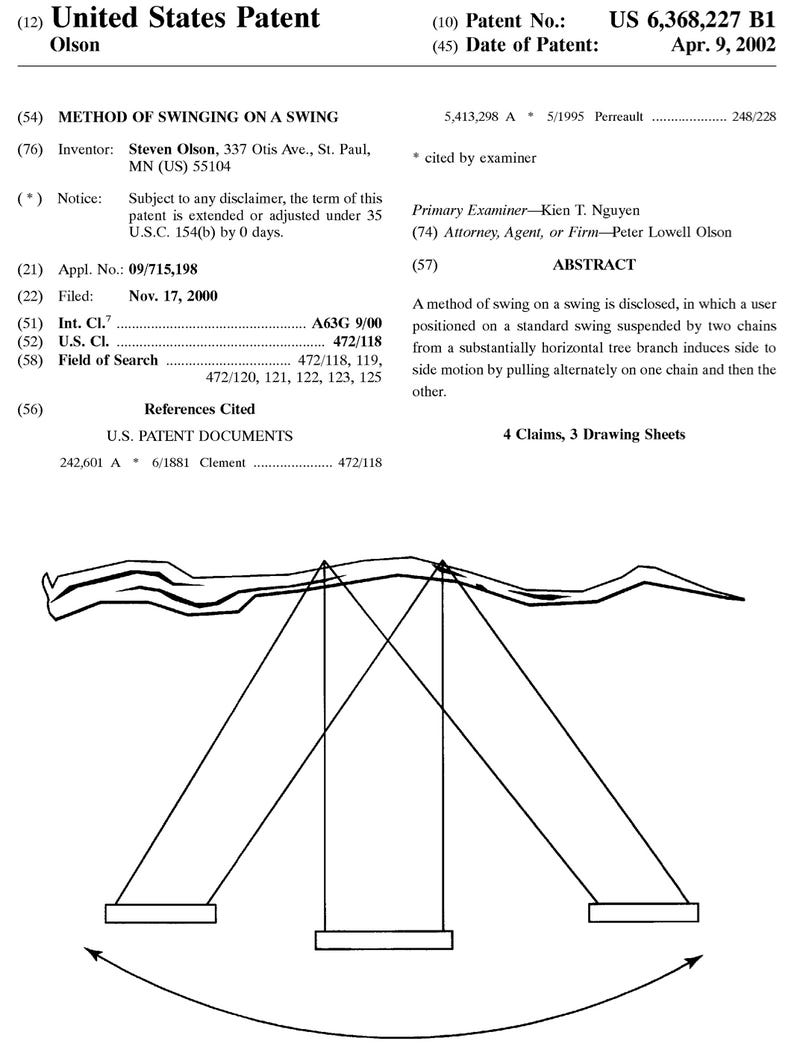
How many patents are inventions?
It’s going to be hard to second-guess the patent office and assess how many patents are assigned to meritorious inventions. But we can explore a related question: how many patents are valuable? If a patent is not valuable, it’s more likely that the underlying invention is not valuable either (though it could also be the invention is valuable, but the patent just does a very bad job of helping you capture that value - in practice manufacturing firms say only about a third of their inventions are well protected by patents).
One place to start is the share of patents where the patent-holder finds it worth their while to pay the fees to keep a patent active. In the USA, as of March 2024, it cost $2,000 to keep your patent in force after 4 years, another $3,760 to keep it in force after 8 years, and another $7,700 to maintain it beyond 12 years (with discounts for small patent-holders). Given those prices, if you choose not to renew your patent, you are implicitly admitting that you do not expect the patent to bring in more than a few hundred to a few thousand dollars per year for the rest of the patent’s life.
It turns out that people frequently find it not worth paying several thousand dollars to keep their patents in force for more years. For example, in Bessen (2008), 20% of patents granted in 1994 were abandoned after just 4 years; almost 60% were abandoned before the end of their term. Arts and Fleming (2017) find somewhat more renewals for a sample of patents ranging from 1981 to 2002, by inventors with more than one invention, but still find a lot of patent holders abandon their patents.3 A lot of other work has reached a similar conclusion: the value of patents is very skewed, with a lot of patents not worth much and and a small number of patents accounting for a large share of total patent value. For example, in a sample of the patents of publicly traded firms from 1926-2010, Kogan et al. (2017) find the average patent received slightly more than 10 citations from other patents (commonly interpreted as a signal of technological significance); but 16% received none, and the top 5% received more than 38. All told, it is plausible that the majority of what patents describe isn’t a big part of technological progress.
But in fact, this issue isn’t as much of a dealbreaker as you might think, because there are a lot of ways to sort the wheat from the chaff, and eliminate many of the junk patents from analysis. One common approach is to restrict attention to “triadic” patents, which are inventions where patent protection has been sought in the big three markets: the USA, Europe, and Japan. Since it is costly to apply for a patent, applying for protection in multiple markets is a signal that the patent-holder believes the patented invention will be worth enough in multiple markets to justify the hassle of application.
Another common approach is to weight patents by the number of citations they receive from other patents. One needs to be careful in how to interpret what a patent citation means (see Measuring Knowledge Spillovers: The Trouble with Patent Citations for full discussion), but a large literature has found the number of citations a patent receives is correlated with other proxies for the value of the patent.4 This might be, for example, because valuable technologies attract copycats, who differentiate their product to the minimum extent necessary to secure a new patent, but who nonetheless cite the original patent (note that if the applicant doesn’t cite a relevant patent, the patent examiner can add it themselves).5
Another common approach is to focus exclusively on the most highly cited patents in a given technology cluster, in a given year. Another approach focuses on the text of patents, looking for those that introduce new technology words and phrases.6 Another approach looks at how the stock market value of publicly traded firms moves when patents are granted.7 None of these approaches are perfect of course, but they all go a long way towards addressing the problem that we probably care a lot more about some of the inventions described by patents than others.
Are patents correlated with other measures of innovation?
But there’s another way to see how serious a problem it is that not all inventions are patented and not all patents are genuine inventions. Instead of starting with inventions and trying to match them to patents, another option is to start with data on patenting and see how well it predicts other measures of innovation. For example, are firms with more patents, or industries with more patents, more innovative by other measures? A lot of papers do this and tend to find that patents (weakly) predict innovation.8
For example, studies find when a firm has more patents, it’s more likely to offer products with new UPC codes. Another study finds that when semiconductor firms have more patents, they are more likely to offer products with improved storage capacity. In yet another study, we observe a similar relationship between rates of technical improvement and the number of patents across 28 different technologies, measuring progress in each technology by whatever metric is appropriate (kilowatt-hours per dollar, lumen-hours per dollar, etc.). Patenting is also correlated with R&D spending.
But there’s an important caveat: as a general rule, the link between patenting and other measures of innovation is a small one. Increase patenting by 10% and you’ll typically see much less than a 10% increase in whatever your measure of innovation you’re using. That makes sense if patents are not covering the majority of inventions, and if a large share of the patents don’t point to valuable inventions.
Indeed, there is also a class of evidence where the link between patenting and an alternative measure of innovation is too shaky to identify, unless you first clean up the patent data using some of the methods discussed above. Compare raw patent counts to total factor productivity growth for firms or industries, and you’ll generally not find a robust association between the two. That echoes the results of the figure we put at the top of this post. But if you work to separate out good and bad patents, studies do tend to find having more valuable patents is pretty robustly associated with faster productivity growth.
Indeed, if you use improved patent data, measures of innovation based on patents and productivity start to line up. To take one example, Kogan et al. (2017) attempted to estimate the dollar value of patents by looking at how the stock price of patent holder’s changes when the patent is granted. Using their estimates of the dollar value of patents, Kogan and coauthors can improve on a simple patent count over time; instead of treating all patents as identical, they can derive the total estimated value of all patents granted in a year. In the following figure, the solid black line is the estimated dollar value of all patents, divided by total GDP in the same year they were granted (and then taking the log of this value). I’ve layered on top of this graph the capital-utilization adjusted TFP growth time series that I kicked off this post with.
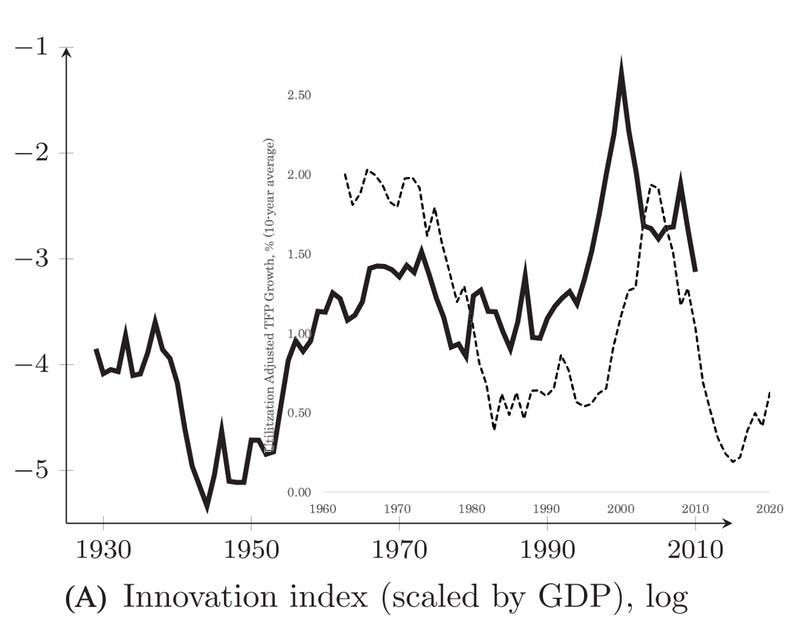
These series match a bit better than the figure comparing raw patent application counts to TFP growth; especially if we think its plausible the patent data will lead the TFP data by a few years, since there will typically be a lag between when a new technology is invented and when it is widely adopted (and hence can affect productivity).
To take one more example, Kalyani (2024) uses text analysis to identify what he calls “creative” patents - those containing an unusually high number of new technical phrases. In the figure below, in green he tracks the change in the number of creative patents (per capita) against decadal-TFP growth rates (green bars). In blue, for comparison, he plots the change in total patents per capita.
As with Kogan et al. (2017), Kalyani’s creative patent series does a better job of matching changes in TFP growth. Both Kalyani (2024) and Kogan et al. (2017) also show these improved patent metrics are associated with TFP growth among individual firms.
So patents are noisy, but especially if we try to filter out some of the noise, they do seem to be correlated with lots of different ways of measuring innovation. And the good news is there are just so many patents; plenty enough for us to do well-powered statistical analyses. So long as patents contain signal, as well as noise, in a big enough sample the signal can shine through and teach us a lot in aggregate. The trouble is not so much that the link between patents and innovation is (very) noisy. It’s that the noise is not random.
Patents are a biased sample
To illustrate the problems of bias, suppose we wanted to know whether one city was more innovative than another - let’s call these cities Metropolis and Gotham. Suppose Metropolis has more patents than Gotham. Does that mean Metropolis is likely to be more innovative than Gotham?
Well, a first issue is that there is substantial variation in the propensity to patent inventions across different technology fields. In a survey of the R&D practices of manufacturers (discussed more here), Cohen, Nelson, and Walsh (2000) found newly invented products are patented about half the time, and newly invented processes less than a third of the time. So even if all industries patent product and process inventions at the same rate, industries where a greater share of technological progress is attributable to process inventions will have a smaller share of technological progress reflected in the patent record. But industries do not patent products and processes at the same rate either. The same survey found the average respondent claims to patent 49% of their product inventions. But for 8 out of 34 industries surveyed the figure was below 33% and for 4 more it was about 66%.
So, if Metropolis has more patents than Gotham, it might merely be that Metropolis is composed of industries with a greater propensity to patent their inventions.
But there are other kinds of bias as well. For example, larger firms appear more likely to patent their inventions. Mezzanotti and Simcoe (2022) finds larger firms are much more likely to rate patents as “important” in the Business R&D and Innovation survey. For example, 69% of firms with more than $1bn in annual sales rate patents as somewhat or very important, compared to just 24% of firms with annual sales below $10mn. This relationship also holds when you compare responses across firms belonging to the same sector, in the same year. Argente et al. (2023) also find that larger firms are likely to have more patents per new product, with the largest firms having roughly twice as many patents per new product as the smallest.9 (Both these papers are discussed in more detail here.)
So, if Metropolis has more patents than Gotham, it might also be that it is the headquarters of more large firms, compared to Gotham, which will tend to patent at a higher rate.
Even looking at firms of a similar size, and belonging to the same industry, there is significant variation in the propensity to patent across firms and across time. Mezzanotti and Simcoe (2022) provide suggestive evidence on this front by computing a measure of “patent intensity”; patents divided by R&D spending. A regression that tries to predict patent intensity with information on the size of a firm and its industry can only explain about 14% of the variance in patent intensity. The rest of the variation is due to firms in the same industry, of roughly the same size, differing in how much they patent per dollar of R&D, and to firms varying across time in how many patents they seek per dollar of R&D.
So, it Metropolis has more patents than Gotham, it could also be down to idiosyncrasies in how the firms in Metropolis think about the value of patenting, relative to firms in Gotham. Maybe they just have a culture of patenting there. In short, if Metropolis has more patents than Gotham, it could be that this is because the city has more innovation going on. But there are several other possibilities as well, and these other factors could swamp genuine differences in the rate of innovation.
These kinds of issues probably help account for the breakdown of the relationship between total US patenting and US total factor productivity growth across time, the relationship plotted in the figure that opened this post. Over the time period covered in the figure, there appears to have been an increase in the share of R&D performed by the biomedical sector10 which is also disproportionately likely to patent its inventions. There has also been an increase in the share of economic activity performed by very large firms who are more likely to patent.11 Additionally, legal changes in the 1980s may have made patenting more attractive for all firms and expanded the set of patentable subject matter (See Kortum and Lerner (1998) for one discussion of these issues). Finally, total factor productivity is itself an imperfect measure of innovation.12
Can Research Correct Biases?
One way to think about this is that a bunch of different factors jointly determine how many patents you observe. One of those factors actually is innovation! But there’s a lot else in there too. So head-to-head counts of patents across firms, cities, industries, or years, is not a great way compare innovation rates.
But head-to-head counts of raw counts is not generally what academic studies are doing. In careful academic work, you can adjust for these various confounds, one-by-one removing the influence of these other factors and leaving behind variation that is mostly driven by changes in innovation. Instead of comparing patenting across all firms and years, break all the firms into their different industries, and then within each industry, compare patenting rates across firms. Better yet, get information on the size of firms, and break all the firms in each industry into different size categories, and compare patent rates across firms that are of similar sizes. And then go a step further and do this exercise year by year.
Better yet, find the average rate of patenting for each firm (to account for differing industrial and firm-level patenting propensities), and find the average rate of patenting for all firms in a particular year (to account for changes in the appeal of patenting). Then look to see when a firm’s patenting is systematically different from its own long-run average, and the average across firms in that year. Those kinds of systematic divergences are more likely to be driven by changes in actual invention, rather than the fact that firms differ in their propensity to patent, or that legal changes (and other factors) may make all firms more or less likely to patent at a particular point in time.
Do all these corrections really work though? Maybe the biases are too subtle for even very careful work to rule out.
One way to check would be to identify settings where the same general phenomena is investigated in multiple ways; sometimes with patents, sometimes with alternative data sources. If the patent data is hopelessly compromised and biases can’t be reliably removed, then we would expect studies using patent data to get different kinds of results than studies that don’t rely on patents. The results of the patent-based studies would be driven by the biases unique to patents, and the results of the non-patent-based studies would be driven by genuine innovation or the (likely different) biases that bedevil the non-patent data.
In Do studies based on patents get different results? I did this kind of analysis for the articles covered in New Things Under the Sun. Across all 37 posts I’ve written that discuss both patent and non-patent evidence, I thought the two approaches basically agreed about 85% of the time. The other 15% of time there was some mix of agreement and disagreement. As discussed in the linked post, there are some potential biases in that analysis, but on the whole I think this suggests careful academic work does generally extract meaningful signal from patent data and evades the biggest sources of bias.
Can we learn from patent data?
To sum up: a large share, likely the majority, of inventions are not part of the patent record. And a large share of patents, possibly the majority, don’t describe important components of technological progress. Other stuff besides invention drives a lot of patenting decisions. But innovation is also in the mix, and so in aggregate, patent data is correlated with other measures of innovation, albeit with a lot of noise. Patents are not good enough to reliably measure innovation without a lot of adjustment. But there are a lot of ways you can analyze patent data to pull out more signal and filter out more noise. Done well, we can learn a lot that appears to be true and useful.
So, can we trust papers built on patent data? In short, I think the answer is yes.
But the longer answer is that social science is hard, we rarely have precise measures of the phenomenon we care about,13 and patents, as used by the academic community, are not much better or worse than anything else you read about in these disciplines. Don’t rely exclusively on patent data, but neither should you rely exclusively on any kind of data in the social sciences. The best we can do is usually see if multiple approaches to a problem converge on similar answers. That’s the premise of this website and what I plan to continue doing.
Thanks for reading! As always, if you want to chat about this post or innovation in generally, let’s grab a virtual coffee. Send me an email at matt.clancy@openphilanthropy.org and we’ll put something in the calendar.
Thanks Eli Dourado for suggesting.
The link goes to a New Things Under the Sun post on this topic, which the following briefly summarizes.
Arts and Fleming only report data on a transformed value of the renewal data (the log of 1 plus the number of renewals). If we use that same transformation on Bessen’s data, we would get an average of 0.91. If everyone renewed their patents at 4 and 8 years, but not 12 years, the transformed data would average 1.09. In fact, Arts and Fleming get an average of 1.05 on their data.
This is a general, but not universal tendency. The “Method for Swinging on a Swing” patent has 22 citations! The majority of these citations have nothing to do with swings and my best guess is they are silent protests against the legitimacy of intellectual property rights. Or maybe jokes.
See Nagaoka, Motohashi, and Goto (2010) for some discussion of these methods.
See e.g., Arts, Hou, and Gomez (2021), Kelly et al. (2021), Kalyani (2024)
See Kogan et al. (2017).
The link goes to a New Things Under the Sun post about this topic, which the following briefly summarizes.
See figure 5 in the linked paper.
Even as large firms might be less innovative per dollar - see The Size of Firms and the Nature of Innovation.
See Vollrath (2020) for a discussion of some non-innovation related factors that explain part of the post-2000 slowdown in TFP growth.
As an innovation economist once said to me, exasperated, when I asked him what he thought about patent data: “it’s not like GDP is perfect!”