Where do new ideas and technologies come from? One school of thought says they are born from novel combinations of pre-existing ideas. It’s an appealing notion, but does this perspective get us anywhere useful? Let’s look at a few papers that have tried to do something useful with this observation.
One nice thing about the combinatorial perspective on innovation, is that if you know all the possible technological building blocks you can exhaustively enumerate all possible inventions, including ones that are never actually invented. For example, once components A, B, and C, exist, then the combination ABC is a possible invention, whether or not anyone has actually “done” it. Then you can see what kinds of factors are correlated with possible inventions being realized.
In Clancy (2017) (yes, that’s me), I used the 13,000+ “mainline subclasses” in the US patent classification system as proxies for the underlying technological “components” of patented innovations. For example, a mainline sub-class might be “Bridge; truss” or “Data processing (artificial intelligence); neural network.” When a patent gets assigned more than one of these, I interpret it as an invention combining these technological components.
I show a patent with a given combination ABC is more likely to be filed in a year when there are more patents combining any of the pairs AB, AC, and BC, but less likely to be filed when there are more patents combining all three (ABC). The following two figures illustrate these dynamics (the different lines correspond to different values for other explanatory variables; i.e., all other variables take the value of the mean, or the mean +/- a standard deviation).
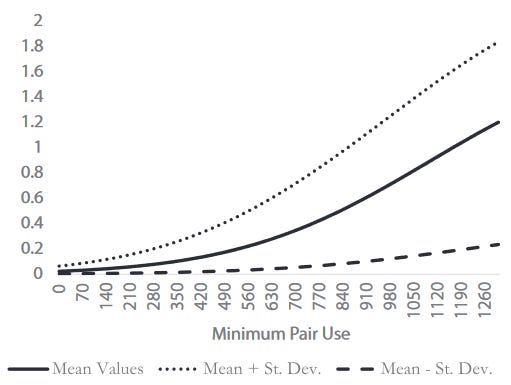
In each figure, we plot the expected annual number of patent applications as we vary the number of times the constituent pairs have been used (above) or the number of times the entire triple-combination has been used (below).
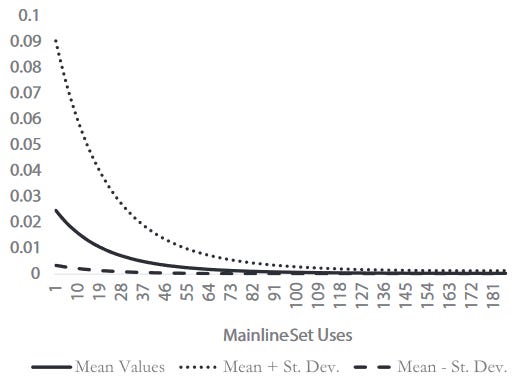
The idea is that inventors learn from the example of successful patents, so anytime they see an invention successfully combining A and B, A and C, or B and C, they have more information about how to combine A, B, and C. Accordingly, it’s more likely such patents will be filed, as in the first figure. On the other hand, patents combining A, B, and C are also instructive, but they also make it harder to patent since it becomes harder to make a novel (patentable) contribution, as in the second figure.
The implication is that innovation enables neighboring ideas that draw on similar but not exactly the same components.
So that’s one non-trivial thing you can do with a combinatorial model. A second nice thing about the combinatorial perspective is it suggests a natural way to measure the novelty and creativity of innovations. A classic paper here is Fleming (2001), which also uses patent subclasses to proxy for combining technologies. For a sample of patents granted in 1990 he calculates the number of prior patents assigned the exact same set of subclasses. He shows patents assigned combinations without much precedent tend to receive more citations, but they’re also riskier, in the sense that they are more likely to get many citations or none at all.
This is sort of what we would expect: compared to incremental improvements, novel innovations have a higher chance of being hits or flops.
Others have applied a similar idea to academic papers. Wang, Veugelers, and Stephan (2017) define highly novel papers as those whose citations “combine” journals not previously connected (weighted by how distant the journals are, in terms of the overlap of journals they are usually cited with). They find all sorts of interesting things about papers that make these novel connections. As with Fleming, they find these papers have a higher variance of citations received, but tend to be more highly cited in the long run.
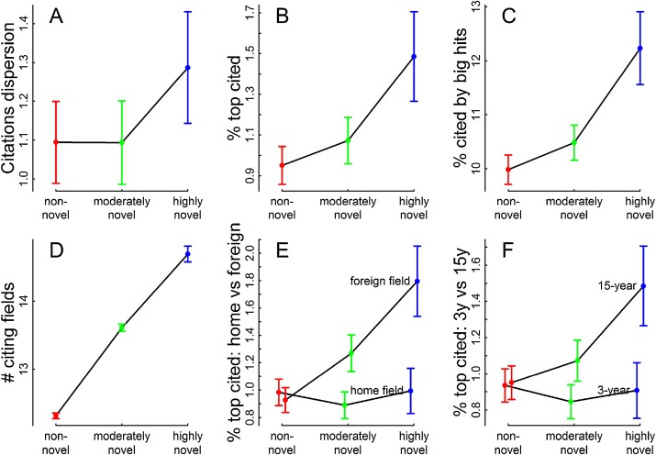
However, they also find evidence of bias against novelty in science: papers making highly novel connections are less likely to appear in high-impact journals, receive a higher share of citations from outside their own field, and citations they do receive take longer to arrive.
Uzzi, Mukherjee, Stringer, and Jones (2013) also looks at papers that make atypical connections between cited journals. They measure the novelty of a combination of journals by the number of times journals have been cited together relative to what would be expected by chance. They also find some ambivalent results about about novelty. The papers most likely to become “hits” (top 5% most highly cited) are those that make a small number of atypical connections but are otherwise highly conventional. Papers that are novel across the board (“low median convention” and “high tail novelty” in the figure below) don’t fare nearly as well.
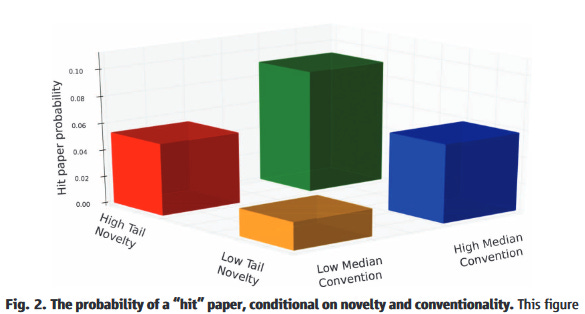
So the observation that innovation is about combining novel ideas isn’t “true-but-useless.” If you have ways of measuring the knowledge building blocks that patents and papers embody, a combinatorial perspective can tell us something interesting we didn’t already know. In the future, I’ll turn to some evidence from beyond the world of papers and patents.
Post-script
Thanks for reading! If you like this, you can help to improve this newsletter by sending me interesting papers on the economics of innovation, especially stuff you think isn’t well known.