Like the rest of New Things Under the Sun, this article will be updated as the state of the academic literature evolves; you can read the latest version here.
Isaac Asimov’s Foundation series imagines a world where there are deep statistical regularities underlying social history, which “psychohistorian” Hari Seldon uses to forecast (and even alter) the long-run trajectory of galactic civilization. Foundation is science fiction, but what if we could develop a model of the “deep laws” governing society? What might they look like?
Well, as a starting principle, we might reflect that in the very long run, material prosperity is driven primarily by technological progress and innovation. So long-run forecasts might depend on the future rate of technological change. Will it accelerate? Slow? Stop?
But this poses a problem. By definition, innovation is the creation of things that are presently unknown. How can we say anything useful about a future trajectory that will depend on things we do not presently know about?
In fact, I think we can say very little about the long-run outlook of technological change, and even less about the exact form such change might take. Psychohistory remains science fiction. But a certain class of models of innovation - models of combinatorial innovation - does provide some insight about how technological progress may look over very long time frames. Let’s have a look.
Strange Dynamics of Combinatorial Innovation
Twenty years ago, the late Martin Weitzman spelled out some of the interesting implications of combinatorial models of innovation. In Weitzman (1998), innovation is a process where two pre-existing ideas or technologies are combined and, if you pour in sufficient R&D resources and get lucky, a new idea or technology is the result. Weitzman’s own example is Edison’s hunt for a suitable material to serve as the filament in the light bulb. Edison combined thousands of different materials with the rest of his lightbulb apparatus before hitting upon a combination that worked. But the lightbulb isn’t special: essentially any idea or technology can also be understood as a novel configuration of pre-existing parts.
An important point is that once you successfully combine two components, the resulting new idea becomes a component you can combine with others. To stretch Weitzman’s lightbulb example, once the lightbulb had been invented, new inventions that use lightbulbs as a technological component could be invented: things like desk lamps, spotlights, headlights, and so on.
That turns out to have a startling implication: combinatorial processes grow slowly until they explode.
Let’s illustrate with an example. Suppose we start with 100 ideas. With a little math, we can show the number of unique pairs of ideas that can be created from these 100 is 4950. But most of these ideas born in this way, from combining all possible ideas, are going to be garbage like “chicken ice cream.” Let’s assume only 1% are viable. Rounding down, our supply of 100 ideas yields 49 new viable ideas (1% of 4950) after extensive R&D to test all possible combinations.
In the next period, we’ll have 149 ideas available. We can go through the whole exercise, calculating how many unique pairs are possible (11,026). Of course, 4950 of those we’ve already investigated. But there are still 6076 new pairs, and if 1% of them are useful, we’ve added 61 more ideas to our stock of ideas. Now we have 210 ideas, and we can go through the process again. If we grind this process forward in time, the number of good ideas in every period of R&D evolves as follows.
Up through period 5, the growth of ideas via this combinatorial process looks roughly like an exponential process. But by the time we get to period 6, an explosion is underway, where the number of new ideas in the next period always becomes so large that it renders the cumulative number of all prior ideas negligible.
Do such processes happen in the real world though? Well, here is an estimated figure of GDP per capita over two millenia. Looks familiar!
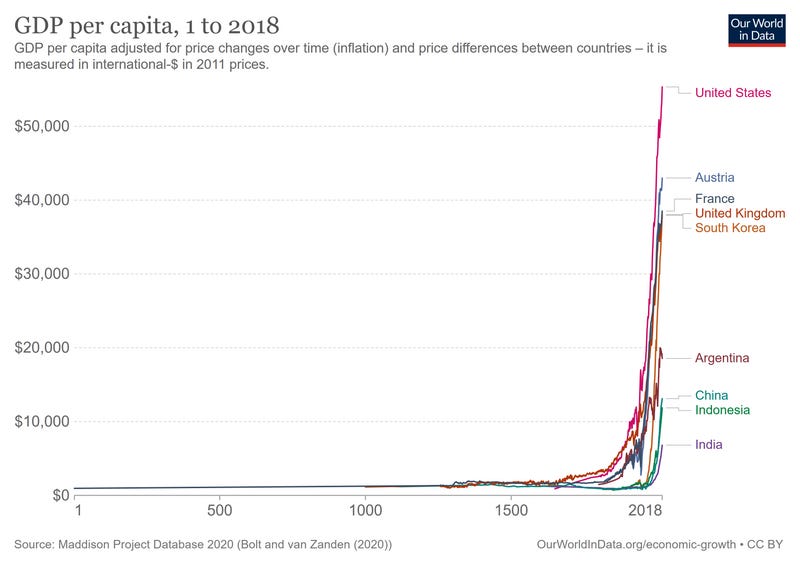
The Industrial Revolution
Weitzman alludes in passing to the fact that combinatorial innovation’s prediction of a long period of slow growth followed by an explosion seems to fit the history of innovation quite well. A 2019 working paper by Koppl, Devereaux, Herriot, and Kauffman expands on this notion as a potential explanation for the industrial revolution. For Weitzman, innovation is a purposeful pairing of two components, but for Koppl, Devereaux, Herriot, and Kauffman, this is modeled as a random evolutionary process, where there is some probability any pair of components results in a new component, a lower probability that triple-combinations result in a new component, a still lower probability that quadruple-combinations result in a new component, and so on. They show this simple process generates the same slow-then-fast growth of technology.
Now; their point is not so much that the ultimate cause of the industrial revolution is now a solved question. They merely show a process where people occasionally combine random sets of created artifacts around them and notice when they yield useful inventions inevitably transitions from slow to very rapid growth. It’s not that this explains why the industrial revolution happened in Great Britain in the 1700-1800s; instead, they argue an industrial revolution was inevitable somewhere at some time, given these dynamics. Also interesting to me is the idea that this might have happened regardless of the institutional environment innovators were working in. If random tinkering is allowed to happen, with or without a profit motive, then you can get a phase-change in the technological trajectory of a society once the set of combinatorial possibilities grows sufficiently large.
The Birth of Exponential Growth
Weitzman and Koppl, Devereaux, Herriot, and Kauffman both show that innovation under a combinatorial process accelerates, which is consistent with a very long run view of technological progress. But it doesn’t seem to fit today’s world. Indeed, for one hundred years, growth in GDP per capita has been remarkably consistent. What’s going on here?
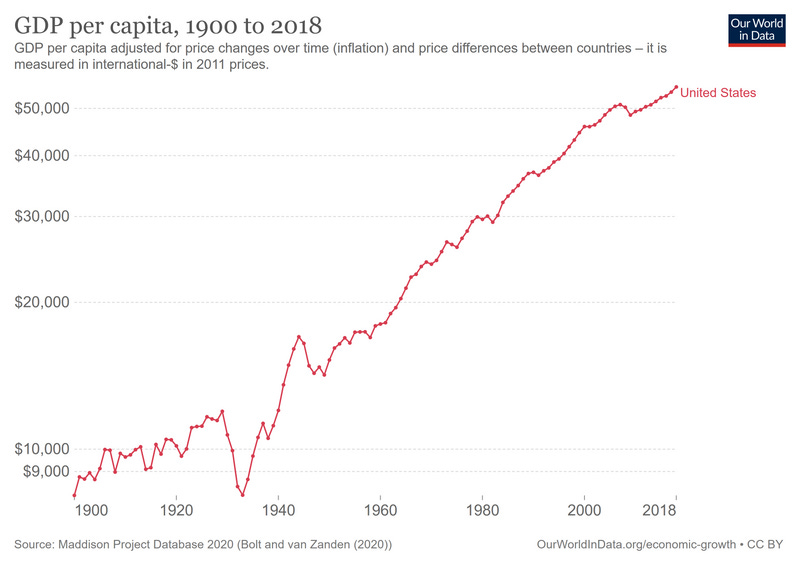
Again, Weitzman proposes a solution. The reason technological progress does not accelerate in all times and places is because in addition to ideas, Weitzman assumes innovation requires R&D effort. In the beginning, we will usually have enough resources to fully fund the investigation of all possible new ideas. So long as that’s true, the number of ideas is the main constraint on the rate of technological progress and we’ll see accelerating technological progress. But in the long run, the number of possible ideas explodes and growth becomes constrained by the resources we have available to devote to R&D, not by the supply of possible ideas.
The basic idea is easy to see. Start with the previous example, but imagine it costs, say, $1mn to combine two ideas and then see if they work out. In 2020 US GDP was $21 trillion, so if the US economy was 100% devoted to R&D it could investigate 21mn ideas per year. Returning to our illustrative example from earlier, that means the US could fully fund R&D on every possible idea for periods 0-5 (that’s not necessarily obvious, but if you do the math, you’ll see in each of these periods there are less than 21mn possible ideas). Indeed, even in period 5, when there are 1.6mn possible ideas to investigate, the US could fully investigate every idea with less than 10% of the economy’s resources. During this time, technological progress would feel like an accelerating exponential process.
But in period 6, there are 153mn possible new ideas, far more than the US has the resources to investigate. From that point going forward, the economy must grow at an exponential rate. The number of ideas has become irrelevant, since there are so many that we’ll never begin to explore them all. All that’s relevant is the amount of resources you can throw at R&D, and that can never be more than 100% of all resources. Think of how an animal population grows at a constant exponential rate when food is not a constraint, because each member of the population spawns a fixed number of children. Just so, an economy grows at a constant rate when the number of ideas is not a constraint, because each technology generates some income, which can be reinvested in R&D to generate a fixed number of “children” technologies.
Why might technological progress be exponential?
But there is a buried assumption in here that we might want to scrutinize. In our illustrative example, we assumed 1% of possible ideas are viable. We don’t need to assume it’s 1%, but if we want to observe constant exponential progress over the very long run in this model, we do need to assume there is some constant proportion of ideas that are viable. And that hardly seems to be guaranteed. Maybe innovation gets harder, for example, because combining ideas that are themselves large hierarchies of combined ideas, get progressively harder to combine. Or maybe innovation gets easier, for example, because eventually we invent things like artificial intelligence to help us efficiently find the viable combinations.
Weitzman explores some of these possibilities, but the best he can really do is establish some possible cases and point to the kinds of criteria that lead to one case or another. If it gets progressively harder to combine ideas, and if ideas are sufficiently important for progress, then growth can halt. On the other hand, if ideas get easier to combine over time then you can get a singularity - infinite growth! And then there are cases where growth remains steady and exponential. That doesn’t help us much to predict the long-run trajectory of society, a la Hari Seldon in the Foundation series.
But Jones (2021) maps out an alternative plausible scenario. So far we have assumed some ideas are “useful” and others are not, and progress is basically about increasing the number of useful ideas. But this is a bit dissatisfying. Ideas vary in how useful they are, not just if they’re useful or not. For example, as a source of light, the candle was certainly a useful invention. So was the light bulb. But it seems weird to say that the main value of a light bulb was that we now had two useful sources of light. Instead, the main value is that light bulbs are a better source of light than candles.
Instead, let’s think of an economy that is composed of lots and lots of distinct activities. Technological progress is about improving the productivity in each of these activities: getting better at supplying light, making food, providing childcare, making movies, etc. As before, we’re going to assume technological progress is combinatorial. But we’re now going to make a different assumption about the utility of different combinations. Instead of just assuming some proportion of ideas are useful and some are not, we’re going to assume all ideas vary in their productivity. Specifically, as an illustrative example, let’s assume the productivity of combinations is distributed according to a normal distribution centered at zero.
This assumption has a few attractive properties. First off, the normal distribution is a pretty common distribution. It’s what you get, for example, if you have a process where we take the average of lots of different random things, each of which might follow some other distribution. If technology is about combining lots of things and harnessing their interactions, then some kind of average over lots of random variables seems like not a bad assumption.
Second, this model naturally builds in the assumption that innovation gets progressively harder, because there are lots of new combinations with productivity a bit better than zero (“low hanging fruit”), but as these get discovered the share of combinations with better productivity get progressively less common. That seems sensible too.
But this model also has a worrying implication. Normal distributions are “thin-tailed.” As productivity gets higher, the difficulty of finding a combination with even higher productivity gets much much harder. For example, suppose productivity for some economic activity is currently zero - basically, we can’t do this activity at all. If we do a little R&D and build a new combination, the probability it will have productivity higher than zero is 1/2. So, on average, we’ll find one useful innovation for every two we explore. If productivity is currently “1”, it gets harder to find a new combination with productivity higher than that. On average, we’ll find one useful innovation for every six we explore. If we grind this forward, we get the following figure, which plots the expected number of combinations that must be explored in order to find one combination with higher productivity than the current best.
By the time we advance to a technology with productivity of 5, on average we’ll have to explore more than 3 million combinations before we find one better!
It looks like we have a process that initially grows very slowly and then explodes. But that’s precisely the shape of a combinatorial explosion as well! Indeed, the point of Jones’ paper is to show these processes balance each other out. Under a range of common probability distributions (such as the standard normal, but also including others), finding a new technology that’s more productive than the current best gets explosively harder over time. However, the range of options we have also grows explosively, and the two offset each other such that we end up with constant exponential technological progress. Which is a pretty close approximation to what we’ve observed over the last 100 years!
This is interesting, but it does conflict with Weitzman’s story of why combinatorial innovation eventually leads to steady exponential growth. In Weitzman, steady growth arose from the fact that doing R&D required some real resources. So even though the set of possible ideas explodes in a way that can offset explosively harder innovation, in Weitzman’s paper it’s not possible to explore all those possibilities because R&D resources are limited. The result is steady exponential growth in Weitzman’s model.
If we had to spend real economic resources to explore possible combinations in Jones’ model, we wouldn’t end up with steady exponential growth. That’s because we would only be able to increase our resources to explore ideas at an exponential rate, but the number of ideas we would need to explore in order to find a technology better than the current best practice increases at a faster than exponential rate. The net result would be slowing technological progress.
But Jones has a different notion of R&D in mind. In Jones’ model, we still need to spend real R&D resources to build new technologies. But it’s sort of a two-stage process, where we costlessly sort through the vast space of possibilities and then proceed to actually conduct R&D only on promising ideas. This way of thinking reminds me an essay on mathematical creation by famed mathematician Henri Poincaré. He describes his two-stage process of creating new math as follows:
One evening, contrary to my custom, I drank black coffee and could not sleep. Ideas rose in crowds; I felt them collide until pairs interlocked, so to speak, making a stable combination. By the next morning I had established the existence of a class of Fuchsian functions, those which come from the hypergeometric series; I had only to write out the results, which took but a few hours.
Poincaré has a two-stage process of invention. He explores the combinatorial space of mathematical ideas, and when he hits on a promising combination, he does the work of writing out the results. But is it really possible for a human mind, even one as good as Poincaré’s, to consider all possible combinations of mathematical ideas?
No. As a mathematician, Poincaré is aware of the fact that the space of possible combinations is astronomical. Mathematical creation is about choosing the right combination of mathematical ideas from the set of possible combinations. But, he notes:
To invent, I have said, is to choose; but the word is perhaps not wholly exact. It makes one think of a purchaser before whom are displayed a large number of samples, and who examines them, one after the other, to make a choice. Here the samples would be so numerous that a whole lifetime would not suffice to examine them. This is not the actual state of things. The sterile combinations do not even present themselves to the mind of the inventor. Never in the field of his consciousness do combinations appear that are not really useful, except some that he rejects but which have to some extent the characteristics of useful combinations. All goes on as if the inventor were an examiner for the second degree who would only have to question the candidates who had passed a previous examination.
So long as we have a way to navigate this vast cosmos of possible technologies and zero in on promising approaches, we can obtain constant exponential growth - even in an environment where pushing the envelope and finding something more useful than the current best practice gets progressively harder.
Long-run Growth and AI
But what if there are limits to this process? Human minds may have some unknown process of organizing combinations, to efficiently sort through them. But there are quite a lot of possible combinations. What if, eventually, it becomes impossible for human minds to efficiently sort through these possibilities? In that case, it would seem that technological progress must slow, possibly a lot.
This is essentially the kind of model developed in Agrawal, McHale, and Oettl (2018). In their model, an individual researcher (or team of researchers) has access to a fraction of all human knowledge, whether because it’s in their head or they can quickly locate the knowledge (for example with a search engine). As a general principle, they assume the more knowledge you have access to, the better it is for innovation.
But as in all the other papers we’ve seen, they assume research teams combine ideas they have access to in order to develop new technologies. And initially, the more possible combinations there are, the more valuable discoveries a research team can make. But unlike in Jones (2021), Agrawal and coauthors build a model where the ability to comb through the set of possible ideas weakens as the set gets progressively larger. Eventually, we end up in a position like Weitzman’s original model, where the set of possibilities is larger than can ever be explored, and so adding more potential combinations no longer matters. Except, in this case, this occurs due to a shortage of cognitive resources, rather than a shortage of economic resources that are necessary for conducting R&D.
As we suspected, they show that as we lose the ability to sort through the space of possible ideas, technological progress slows (though never stops in their particular model).
But if the problem here is we eventually run out of cognitive resources, then what if we can augment our resources with artificial intelligence?
Agrawal and coauthors are skeptical this problem can be overcome with artificial intelligence, at least in the long run. They argue convincingly that no matter how good an AI might be, there is always a number of components where it becomes implausible for a super intelligence to search through all possible combinations efficiently. If that’s true, then in the long run any acceleration in technological progress driven by the combinatorial explosion must eventually stop when our cognitive resources lose the ability to keep up with it.
To illustrate the probable difficulty of searching through all possible combinations of ideas, let’s think about a big number: 10^80. That’s about how many atoms there are in the observable universe. That would seem like a difficult number of atoms for an artificial intelligence to efficiently search over. Yet if we have just 266 ideas, then the number of possible combinations is about equal to 10^80, i.e., the number of atoms in the universe!
Still, maybe there are ways for the AI to efficiently organize this gigantic set, so that it does not actually have to search through more than a tiny sliver of the combinations?
The trouble is, the number of ideas we have available must be much, much larger than 266. As a starting count, we could turn to the US Patent and Trademark Office’s technology classification system, which attempts to classify patents into distinct technological categories. It has about 450 “three digit” classifications, but these categories seem to broad to be much use to an inventor. They include categories like “bridges” or “artificial intelligence: neural networks.” If we instead turn to a more detailed set of classifications, there are over 150,000 subclassifications which get quite specific. If we were to take that as our measure of the number of possible combinations, then we’re talking about 10^45,181 possible combinations of technology. If you took every atom in the universe and stuffed another universe worth of atoms inside each atoms, and then did that again with every one of those new atoms, and then repeated that process in a nesting structure 500 levels deep, you would be getting in the ballpark of how many combinations there are to consider. Seems challenging?
That said, the point is not that an AI can’t accelerate innovation. Perhaps there are domains where the number of possible combinations is larger than human minds can search but not artificial ones. In such domains, AI could accelerate innovation for awhile, via the combinatorial approach. But they would argue, eventually, any given domain would probably accrue too many ideas for any supercomputer to search through all possible combinations.
And lastly, there is more to innovation than searching through combinations. Agrawal and coauthors also point out that AI can serve like a recommender, helping you access more knowledge, and in their model it’s always good to have access to more ideas (even if you aren’t going to be searching through all combinations). I think of it as more like the ability to access a really good google for existing knowledge, so you can borrow and adapt solutions developed elsewhere. In their model, the ability of AI to put you in touch with more of knowledge that’s out there, the better it is for innovation in the short run and as far into the future as you care to go.
Psychohistorians of Technological Progress
To return to the motivation for this article: combinatorial dynamics are a plausible candidate for a deep law of innovation. Those dynamics will tend to deliver slow growth that accelerates for a time, as the combinatorial explosion heats up. But beyond that, two kinds of process may work to stymie continued explosive growth.
Innovation might get harder. If the productivity of future inventions are like draws from some thin-tailed distribution (possibly a normal distribution), then finding better ways of doing things gets so hard so fast that this difficulty offsets the explosive force of combinatorial growth.
Exploring possible combinations might take resources. These resources might be cognitive or actual economic resources. But either way, while the space of ideas can grow combinatorially, the set of resources available for exploration probably can’t (at least, it hasn’t for a long while).
If I can get a bit speculative, ultimately, these ideas seem linked to me. To start, it seems to me that it must take resources to explore the space of possible ideas, whether those resources are cognitive or economic. It may be that, we are still in an era where human minds can efficiently organize and tag ideas in the combinatorial space so that we can search it efficiently. But I suspect, even if that’s true, it can’t last forever. Eventually the world gets too complicated. That suggests the ultimate rate of technological progress depends on how rapidly we can increase our resources for exploring the space of ideas. (If we need more cognitive resources, then that means resources in the form of artifical intelligence and better computers). And our ability to increase our resources with new ideas is a question that falls squarely in the domain of Jones (2021): how productive are new ideas?
If the productivity of new ideas follows a thin-tailed distribution, then we would seem to be in trouble: according to Jones (2021) only so long as we can hunt through the combinatorial landscape are we able to increase productivity at an exponential rate. But an exponential rate isn’t good enough if the resources needed to explore a combinatorial space grow at a faster-than-exponential rate (which they must if they’re proportional to the number of possible ideas). In that case, it would seem that technological progress would need to slow down over time.
On the other hand, suppose the productivity of new ideas follows a fat-tailed distribution. That’s a world where extremely productive technologies - the kind that would be weird outliers in a thin-tailed world - are not that uncommon to discover. Well, in that world, Jones (2021) shows that the growth rate of the economy will be faster than exponential, at least so long as it can efficiently search all possible combinations of ideas. And faster than exponential growth in resources is precisely what we would need to keep exploring the growing combinatorial space.
Which outcome is more likely? Well, the future has no obligation to look like the past, but given that innovation seems to be getting harder, I am hoping for the best but bracing for the worst.
Thanks for reading! If you liked this, you might also enjoy these much more data driven articles arguing that innovation has tended to get harder, and that this might be because of the rising burden of knowledge. For other related articles by me, follow the links listed at the bottom of the article’s page on New Things Under the Sun (.com). And to keep up with what’s new on the site, of course…
Combinatorial innovation and technological progress in the very long run